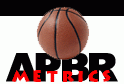 |
APBRmetrics The statistical revolution will not be televised.
|
View previous topic :: View next topic |
Author |
Message |
mtamada
Joined: 28 Jan 2005 Posts: 377
|
Posted: Wed Sep 30, 2009 7:14 am Post subject: Wayne Winston's presentation at NESSIS |
|
|
Here's a report on NESSIS, focusing on Wayne Winston's presentation about his and Sagarin's version of adjusted plus-minus (WinVal).
When the publicity for WinVal first came out, with the descriptions of how it accounted for the ability of the other 9 players on the court, I assumed that Winston and Sagarin were looking at all 450-odd NBA players by solving a system of 450 equations with 450 variables. But it turns out that they run a least squares regression, at its heart the same as DanR's adjusted +/-.
Winston highlighted two ways in which his APM differs from what unnamed others who use OLS might get. He noted that there can be high degrees of collinearity in the data, and said that he has a "proprietary fix for this". More on this later.
He also said that his technique for dealing with players with little playing time differs ... I haven't done APM myself nor looked in detail at how DanR, AaronB, etc. do it but Winston said that some people treat those players as generic replacement level players (I presume in order to get the estimation to run). Winston said he does not do this (but gave no details about what he does do), which can cause some player's results to differ markedly (Dirk Nowitzki changing from -1 to +7).
I think it's fair to say that many in the audience thought that Winston seems overly sure about his results, and seems not to pay attention to standard errors. Some of the questions after his presentation directly or indirectly addressed this, but he IMO dismissed them with hand-waving. E.g. one of his startling results was that Lamar Odom had a better APM in the 2009 playoffs than Kobe did. But I worry about sample size: over a few games, entire teams might, for random reasons, score more or fewer points than they "should" against a given opponent. Individual player's estimated APMs would be subject to even more random variability, i.e. high standard errors.
Slightly different but related to this: Winston acknowledged the debate about whether to use one season or more than one season of data when coming up with APM estimates. Given his predilections above, it's not surprising that he opts to use one season of data.
A comment on collinearity: no surprise that it's there given NBA substitution patterns, and it is a common bugaboo in doing linear regression. Econometricians will tell you that there is only one true solution: get more data (i.e. increase the sample size). Kind of hard because we can't ask the players to go out and play some more games for us (but we can opt to use 2 or even 3 seasons of data, which was the reason I voted for AaronB to use multiple seasons on bbval.com, though I knew the masses would ask for APMs based on one season).
Other solutions? One common one is ridge regression, a weird-sounding technique when you read about how to do it, but it makes sense when you think of it as a Bayesian estimator where, in the face of regression coefficients with unreliable estimates (due to the collinearity), we opt to bias the estimates to zero, similar to regressing estimates to the mean but in this case we regress them to zero.
But my guess is that Winston isn't doing something like that. What is he doing? No idea. Another common suggestion is to transform one or more of the variables, but there's not much transforming that one can do with dummy variables.
So Winston's APM is similar to the other APMs out there, but with some modifications. Do these modifications result in much smaller standard errors? It'd be hard to imagine how they could, but Winston didn't provide standard errors nor address them much in the Question & Answer period.
He did also mention that he's been focusing more on lineups than on individual player ratings, consistent with some of the recent conversations and interests in this group.
The conference concluded with a panel discussion with MikeZ, AaronB, and Ken Catanella from the NBA Office. Ken said that the highly detailed video data that will soon be produced by the NBA will eventually be made available to the public. The panelists gave some interesting descriptions about their work situations, and also some useful advice about getting a job in the NBA -- but most of that same advice has I think already been mentioned on this site.
The final highlight was finally meeting MikeZ, who'd reserved the top floor of a sports bar across the street from where the Celtics play (I refuse to learn whatever dumb corporate name they've given to what should be called the Boston Garden). So after the conference a good proportion of the attendees went there and I got to spend time over food and drinks with him, AaronB, EdK, EliW, and Ben Alamar (editor of JQAS; I hadn't realized that he'd also consulted for the Sonics/Thunder). Swell group of fellows. |
|
Back to top |
|
 |
Ryan J. Parker
Joined: 23 Mar 2007 Posts: 711 Location: Raleigh, NC
|
Posted: Wed Sep 30, 2009 8:49 am Post subject: |
|
|
Thanks for the recap!
My sense is that there is only so much we can squeeze out of the adj +/- model as currently specified. No idea what he could be doing to deal with the collinearity issues, but I think that there is still only so much confidence you can get out of the model. Although multiple years of data reduces std error, I have reservations about what we assume when we do this (not assumptions in the statistical/model sense, but in the basketball sense).
Great stuff, and I hope there will be video posted online! _________________ I am a basketball geek. |
|
Back to top |
|
 |
John Hollinger
Joined: 14 Feb 2005 Posts: 175
|
Posted: Thu Oct 01, 2009 10:51 am Post subject: |
|
|
"Post" entertainment at the Fours has rapidly become a rather enjoyable tradition at the Boston events ... wish I could have been there but the calendar wouldn't let me. |
|
Back to top |
|
 |
mikez
Joined: 14 Mar 2005 Posts: 75
|
Posted: Thu Oct 01, 2009 3:46 pm Post subject: |
|
|
Simply put, something is wrong with you if you don't love the Fours.
Anyways, it was great to see everyone, and especially to finally meet mtamada. Hopefully we'll all do it again in two years (which is how often the organizers have said they plan to do NESSIS).
-MZ |
|
Back to top |
|
 |
mtamada
Joined: 28 Jan 2005 Posts: 377
|
Posted: Thu Oct 01, 2009 4:05 pm Post subject: |
|
|
Correcting something that I wrote:
Quote: | So Winston's APM is similar to the other APMs out there, but with some modifications. Do these modifications result in much smaller standard errors? It'd be hard to imagine how they could |
Actually I should have said that his standard errors may indeed be reduced, but it's hard to imagine that he's reduced them by more, or much more, than other standard techniques such as ridge regression manage to do.
The whole point of ridge regression is to reduce the standard errors of the coefficient estimates, by purposely biasing the estimates (ie. doing that weird thing of adding an artificial number to the X'X data matrix). Usually we want to avoid using biased estimators, but sometimes a biased estimator can have such a good (i.e. smaller) standard error that it's worthwhile to do so: most (all?) of the estimators which utilize regression to the mean (or to zero in this case) are examples.
If Winston has come up with a technique which reduces standard errors and does it substantially better than other techniques, that'd be a major advance and he could publish it in an economics or statistics journal and get accolades aplenty. Maybe he has made such an advance, but wants to keep it proprietary so he can keep raking in the 6-figure consulting fees from the Mavs. More likely I think is that he twiddles with the figures somewhat, possibly in a way which makes sense for NBA data, but is not generalizeable to other data sets, which may indeed yield smaller standard errors but my guess is not by a substantial amount. |
|
Back to top |
|
 |
Ed Küpfer
Joined: 30 Dec 2004 Posts: 787 Location: Toronto
|
Posted: Thu Oct 01, 2009 5:16 pm Post subject: |
|
|
I think I speak for everyone when I say it was great meeting me. _________________ ed |
|
Back to top |
|
 |
mikez
Joined: 14 Mar 2005 Posts: 75
|
Posted: Thu Oct 01, 2009 5:36 pm Post subject: |
|
|
Quote: | I think I speak for everyone when I say it was great meeting me. |
Well, I would've said "seeing" you, but yeah, that's true. However, I'm more looking forward to seeing that beard when it reaches ZZ Top lengths (and I'm not talking about their drummer Frank Beard, who doesn't have one).
-MZ |
|
Back to top |
|
 |
HoopStudies
Joined: 30 Dec 2004 Posts: 706 Location: Near Philadelphia, PA
|
Posted: Fri Oct 02, 2009 9:23 am Post subject: |
|
|
I know there was other basketball work besides Winston's stuff at NESSIS. Can someone give some info on how those were? I've read the abstracts... _________________ Dean Oliver
Author, Basketball on Paper
The postings are my own & don't necess represent positions, strategies or opinions of employers. |
|
Back to top |
|
 |
mtamada
Joined: 28 Jan 2005 Posts: 377
|
Posted: Fri Oct 02, 2009 4:26 pm Post subject: |
|
|
HoopStudies wrote: | I know there was other basketball work besides Winston's stuff at NESSIS. Can someone give some info on how those were? I've read the abstracts... |
There were two basketball-related posters, but I looked at them only briefly (because I was concentrating on a few other posters, plus conversation).
There was a March Madness Tournament poster, they had an interesting and potentially important empirical result (#10 and #11 seeds typically advance further in the tournament than #8 and #9 seeds do, presumably because if they upset their 1st round opponent, they face an easier 2nd round opponent than the hapless #8 and #9 seeds face). But: they are less likely to advance out of the 1st round at all, so I think a proper analysis would look at the balance of advancing to the 2nd round with greater frequency compared to advancing to the 3rd round with greater frequency. Also was this just a random blip or is it inherent in the design, I don't recall seeing a signficance level or standard error (it may've been there and I overlooked it, I only looked at the poster a little bit -- I'm a lot more of a pro basketball fan than college basketball, and this poster had almost no application to the NBA).
The Harvard Sports Analysis Collective (an undergraduate student club -- how many colleges have a sports analysis club?) had a poster evaluating NBA coaches and their impact on team success. The abstract mentioned a lot of potentially interesting topics, but the poster seemed to focus on team's won-loss record relative to their Pythagorean prediction. Which is a nice thing to look at but not earth-shaking. IIRC they also had some exhibits showing that 2-for-1 is a good strategy, and measuring how often coaches did it (not often enough, is their conclusion, again IIRC). That is more innovating and interesting stuff, but I didn't stick around to look at it in detail. They may've had yet other exhibits that examined the other topics raised in their abstract, but I didn't look -- I think at that point I may've gotten distracted by finally spotting EdK or some such.
Carl Morris of Harvard had a poster on uses of odds ratio models in sports, which his abstract says includes basketball. His exhibits were in small print though and I didn't get an up-close look. The abstract suggests that he was showing illustrations of where odds ratio models can be used, which by itself would be nice but not earth-shaking. But they may very well have contained some earth-shaking new stuff, but I didn't get a close look.
With two conferences now, I think some institutional patterns have begun to emerge. Harvard of course produces the most posters, it's their homecourt plus there's that Sports Analysis Collective. The Wharton School seems to produce the 2nd most at least if we also count presentations, this includes both profs and students (mainly grad students I think but I'm not sure). After that, I think a peloton, maybe with Columbia towards the front? And some small colleges in there too, Macalester e.g. A bit surprising that there's not more of an MIT presence (though at least a couple of APBRmetrics members are alumni), maybe they save their stuff for the Sloan Conference? |
|
Back to top |
|
 |
gabefarkas
Joined: 31 Dec 2004 Posts: 1313 Location: Durham, NC
|
Posted: Mon Oct 05, 2009 7:32 pm Post subject: |
|
|
mikez wrote: | Quote: | I think I speak for everyone when I say it was great meeting me. |
Well, I would've said "seeing" you, but yeah, that's true. However, I'm more looking forward to seeing that beard when it reaches ZZ Top lengths (and I'm not talking about their drummer Frank Beard, who doesn't have one).
-MZ |
Who are you so wise in the ways of ZZ Top trivia/minutiae? |
|
Back to top |
|
 |
tpryan
Joined: 11 Feb 2005 Posts: 100
|
Posted: Tue Oct 06, 2009 1:20 am Post subject: |
|
|
mtamada wrote: | Correcting something that I wrote:
Quote: | So Winston's APM is similar to the other APMs out there, but with some modifications. Do these modifications result in much smaller standard errors? It'd be hard to imagine how they could |
Actually I should have said that his standard errors may indeed be reduced, but it's hard to imagine that he's reduced them by more, or much more, than other standard techniques such as ridge regression manage to do.
The whole point of ridge regression is to reduce the standard errors of the coefficient estimates, by purposely biasing the estimates (ie. doing that weird thing of adding an artificial number to the X'X data matrix). Usually we want to avoid using biased estimators, but sometimes a biased estimator can have such a good (i.e. smaller) standard error that it's worthwhile to do so: most (all?) of the estimators which utilize regression to the mean (or to zero in this case) are examples.
If Winston has come up with a technique which reduces standard errors and does it substantially better than other techniques, that'd be a major advance and he could publish it in an economics or statistics journal and get accolades aplenty. Maybe he has made such an advance, but wants to keep it proprietary so he can keep raking in the 6-figure consulting fees from the Mavs. More likely I think is that he twiddles with the figures somewhat, possibly in a way which makes sense for NBA data, but is not generalizeable to other data sets, which may indeed yield smaller standard errors but my guess is not by a substantial amount. |
The original motivation of ridge regression was that the mean square error of estimation could be reduced, perhaps substantially, by introducing some bias while simultaneously greatly reducing the variance. A companion result, due to Theobald, is that the mean square error of prediction can also be reduced.
To clarify a bit, a number is added to each diagonal element of X'X. In ordinary ridge regression the same number is added, whereas in generalized ridge regression a different number is added to each diagonal element.
There are several ways to motivate the use of ridge regression.
From a Bayesian perspective, weak prior information is being introduced, namely that the beta vector does not have infinite length, whereas infinite length is the implicit assumption when OLS is used.
I think the primary motivation for ridge regression, however, should be that the regression coefficients start going crazy in the presence of extreme multicollinearity and become meaningless when interpreted the way that most people interpret them. |
|
Back to top |
|
 |
MoonbeamLevels
Joined: 09 Apr 2007 Posts: 10
|
Posted: Tue Oct 06, 2009 8:34 am Post subject: |
|
|
I'm a PhD student in statistics, and it's fascinating to hear topics such as Ridge Regression emerge here! It (along with other penalized regression models like the LASSO) plays with the concept of the "bias-variance" tradeoff, essentially reducing the variance significantly at the expense of a little bias. Different shrinkage methods have different advantages- the LASSO simultaneously generates parameter estimates while handling the model selection issue by shrinking the parameters toward zero (and setting a number of them equal to zero, in most cases), while Ridge Regression shrinks the coefficients in a way that circumvents collinearity, but does not necessarily set them equal to zero. A useful combination of these two methods, called the "elastic net", seems to be quite promising according to Statistics literature. I'm not sure how well these methods would translate to basketball data, but I would imagine they could indeed be quite useful. |
|
Back to top |
|
 |
HoopStudies
Joined: 30 Dec 2004 Posts: 706 Location: Near Philadelphia, PA
|
Posted: Tue Oct 06, 2009 1:36 pm Post subject: |
|
|
Good to see some of the people with that more advanced stat knowledge providing the input. I didn't know about ridge regression until this discussion. Relating it to Bayesian stuff is definitely helpful... So many tools and you have to know when to use which ones... _________________ Dean Oliver
Author, Basketball on Paper
The postings are my own & don't necess represent positions, strategies or opinions of employers. |
|
Back to top |
|
 |
mtamada
Joined: 28 Jan 2005 Posts: 377
|
Posted: Tue Oct 06, 2009 3:27 pm Post subject: |
|
|
HoopStudies wrote: | Good to see some of the people with that more advanced stat knowledge providing the input. I didn't know about ridge regression until this discussion. Relating it to Bayesian stuff is definitely helpful... So many tools and you have to know when to use which ones... |
That LASSO and elastic net stuff sounds interesting too, with a variety of techniques available one could easily imagine an individual researcher such as Winston mixing a variety of techniques to come up with his estimates.
Even more interesting to me is the stuff that RyanP did and put on his blog last month (and mentioned in the other Wayne Winston thread), with his hierarchical modelling stuff. I've only glanced at it but it looks like RyanP switched from a fixed effects to a random effects model? A potentially substantial step in improving the regression results, and his emprical results suggest that that is indeed the case. Possibilities for shrinking the estimates toward zero are commonly built into these models. One pitfall however is that certain random effects have to meet assumptions about being independent from other random effects. A standard safeguard is to do a Hausman model speficiation test; if it fails then you have to fall back on the fixed effects model. |
|
Back to top |
|
 |
Ryan J. Parker
Joined: 23 Mar 2007 Posts: 711 Location: Raleigh, NC
|
Posted: Tue Oct 06, 2009 4:31 pm Post subject: |
|
|
Thanks for that link mtamada. I haven't seen much work in trying to look at the underlying assumptions and testing if they work or not. In fact, I haven't seen any such talk about when you'd have to revert back to a fixed effects model! _________________ I am a basketball geek. |
|
Back to top |
|
 |
|
|
You cannot post new topics in this forum You cannot reply to topics in this forum You cannot edit your posts in this forum You cannot delete your posts in this forum You cannot vote in polls in this forum
|
Powered by phpBB © 2001, 2005 phpBB Group
|