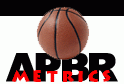 |
APBRmetrics The statistical revolution will not be televised.
|
View previous topic :: View next topic |
Author |
Message |
Dan Rosenbaum
Joined: 03 Jan 2005 Posts: 541 Location: Greensboro, North Carolina
|
Posted: Thu Jul 27, 2006 5:32 pm Post subject: Wins Produced - Wages of Wins (Berri, Schmidt, and Brook) |
|
|
Dave Berri, Martin Schmidt, and Stacey Brook have created a measure called Wins Produced.
http://www.wagesofwins.com/
This measure is a variant of the many linear weights measures out there, but instead of using only theory to come up with the weights, they use both theory and regression techniques.
[This is clearly a working post, as I am making some changes as I reread parts of the book.]
(1) Their first step is to regress team wins onto offensive and defensive efficiency (or pace-adjusted points scored and points given up). What this does, in essence, is give them their weights for points scored, which is 0.033.
(2) Possessions = FGA + 0.44*FTA - OREB + TO = DREB + FGM + 0.44*FTM + TO. So from this and the fact that over their time period teams averaged 1.02 points per possession, they get their multiplier for possessions given up and acquired, which is 1.02 * 0.033 = 0.034. So rebounds, turnovers, and true shot attempts (FGA + 0.44*FTA) get weights of 0.034 or -0.034. Steals also get a multiplier of 0.034 because they are the opposite of a turnover.
(3) Next, they need to estimate the value of assists, blocks, and personal fouls. Let's start with blocks. They estimate that each block reduces opponents' two-point field goals made by 0.65 and since they have already estimated the value of a made two-point field goal (two points minus a field goal attempt) at 0.033, then blocks are worth 0.65*0.033 = 0.021.
(4) Next, they calculate, on average, how many opponents' free throws made are generated by a personal foul. This results in them weighting each personal foul by -0.018.
(5) Now onto assists. They admit that they almost wrote the book leaving assists out altogether. And one reason why they might have considered this is that accounting for assists will only hurt them when go back and see how well their measure predicts wins (they make an adjustment to fix this). But they found that when using wins produced from a previous season to predict wins, assists were a helpful predictor for what they could not explain. Thus, after some work they ended up with a weight of 0.022 for assists.
(6) Then they adjust their Wins Produced measure so that, on average, it is equal for every position. There is no basis for this adjustment in the numbers; they justify it with an argument that a team could not play all centers. (True, but it could be that centers should be paid more.) This adjustment likely will make it harder for them to predict team wins, so that is not a justification for this adjustment.
This adjustment is a big deal in their ratings, and given how critical they are in the book of the understanding basketball people have of the game, it is surprising to see them so comfortable relying on the same kind thinking to justify a pretty big adjustment. And I say this even though I agree with the need for this adjustment.
(7) Lastly, they adjust using team defensive measures. If we think back in terms of the original regression equation regressing wins onto points scored and points given up (per possession), when they aggregate up their Wins Produced by team, they already have accounted for points scored and (most of) possessions. But for points given up, they have only accounted for free throws made (through personal fouls) and part of two-point field goals made (through blocks). So if they account for the rest of two-point field goals made and for three-pointers made, they will have accounted for points given up. They make another adjustment that, in essence, does that. (It also cleans up possessions by accounting for team rebounds and opponents' non-steal turnovers.)
They say in the book that this adjustment barely affects the relative ratings of the players; the correlation between the ratings with and without the adjustment is 0.99.
But my guess is that it is huge in terms of helping them predict wins later on. My guess is that instead of predicting 95% of team wins like they do with this adjustment, they predict less than 70% without it. So that begs the question. If this adjustment barely budges their Wins Produced measure for individual players but is so critical in explaining team wins, it raises a big red flag as to the validity of using the prediction of team wins as a barometer of their methodology.
(8) So at the end of the day when they add up their Wins Produced team by team, what they are in essence doing is predicting points scored and points given up.
To make that point more clearly, I ran a regression of wins onto points scored and points given up. When I do so my predictions of team wins are, on average, 2.4 wins away from actual wins over the 1995-96 through 2004-05 seasons and 1.7 wins away in the 2003-04 season that the book highlights. (These are the same deviations they report in the book for their Wins Produced measure.)
Below I give how far off my predictions are (versus actual wins) and how far off the book's predictions are. (The book's are first, mine are second.) The correlation here is 0.96, so this it is clear that Berri and his co-authors have successfully produced a measure that aggregates up to predict offensive efficiency and defensive efficiency.
Code: | Team Book Mine
ATL 0.55 0.24
BOS 0.82 1.06
CHI 0.70 0.79
CLE 1.06 1.22
DAL 1.15 0.81
DEN 0.73 0.86
DET 2.75 3.67
GSW 2.10 1.92
HOU 0.90 0.92
IND 4.41 3.51
LAC 0.53 0.35
LAL 4.31 4.46
MEM 2.23 2.38
MIA 0.28 0.49
MIL 2.85 2.83
MIN 2.21 1.55
NJN 0.52 1.06
NOH 0.26 0.29
NYK 1.89 2.13
ORL 0.96 0.64
PHI 1.27 0.97
PHO 1.64 1.82
POR 3.10 3.69
SAC 0.38 0.54
SAS 3.44 4.40
SEA 2.31 2.15
TOR 0.09 0.59
UTA 4.41 4.53
WAS 0.68 0.74 |
But all of this still begs bigger questions.
(i) Does an analysis of how team statistics predict wins (which in essence is what Wins Produced does) tell us much about how to use statistics to apportion credit among players on a team?
One of the implications of this approach is that there is no room for credit to be given for shot creation. The authors do not arrive at this assumption empirically; it is simply something they assume given their approach.
In my opinion, understanding the value of creating shots is perhaps the most important aspect of analyzing basketball statistics. If, like in baseball, players each got a turn to take their shot, then this would not be an issue. But that is not the case, so I have a hard time making sense of an approach that assumes away what I consider to be a critical aspect of the game of basketball.
(ii) Remember that without the team adjustments (which have practically no effect on the relative ratings of players), Wins Produced likely does a terrible job predicting team wins. So what this says is that two versions of Wins Produced that are practically identical, one does a great job predicting team wins and the other does a pretty lousy job. What does this say about using the prediction of team wins as a barometer?
(iii) This is not really a criticism of Berri and his co-authors, but I have always felt that our box score statistics tell us more than we give them credit for. For the most part, this book follows the typical approach in logically relating the values of a point scored, field goal missed, rebound, turnover, etc. But I have always felt that these stats also tell us something about players in addition to the impact on the game at the time they occurred. Could guys who turn the ball over or a lot not be as good help defenders? Might the guy who gets steals do a better job keeping the floor spaced? Might the great rebounder do a better job catching tough pasess or picking up loose balls?
This is the logic I have used in relating my adjusted plus/minus ratings to points, rebounds, assists, steals, etc. And I have tended to find that the weights for these stats differ a lot from the logic-based approaches of Dean Oliver, John Hollinger, and Berri and his co-authors.
Last edited by Dan Rosenbaum on Fri Jul 28, 2006 10:05 pm; edited 2 times in total |
|
Back to top |
|
 |
Dan Rosenbaum
Joined: 03 Jan 2005 Posts: 541 Location: Greensboro, North Carolina
|
Posted: Thu Jul 27, 2006 11:48 pm Post subject: |
|
|
This is something that I suspect will spark some conversation. Except for the team adjustments (which shouldn't matter much for this), I approximated Wins Produced, Win Score, and lots of other measures and correlated them to my adjusted plus minus ratings. Here are the results.
[Note I had a typo in one of my formulas that I fixed, resulting in some new correlations relative to those I first reported.]
Wins Produced (no position adjustment): 0.3296
Wins Produced (position adjustment): 0.4545
Win Score (no position adjustment): 0.3079
Win Score (position adjustment): 0.4466
Old Win Score (no position adjustment): 0.1538
NBA Efficiency (no position adjustment): 0.3765
NBA Efficiency (position adjustment): 0.4460
PER (no position adjustment): 0.4345
PER (position adjustment): 0.4423
Offensive minus Defensive Rating (no position adjustment): 0.4137
Offensive minus Defensive Rating (position adjustment): 0.4481
Win Shares (no position adjustment): 0.4897
Win Shares (position adjustment): 0.4863
Win Shares per Minute (no position adjustment): 0.4327
Win Shares per Minute (position adjustment): 0.4420
My Statistical Adjusted Plus/Minus Rating: 0.5820
This is really interesting. Both Win Score and Wins Produced are both pretty terrible without position adjustments, but with the position adjustment they are not bad. It suggests that a lot of other methods have overvalued shot creation. But notice that the position adjustment is really important here - much more important than with any of the other metrics except for NBA Efficiency.
(Berri and co-authors have published at least one paper using what I call the Old Win Score, which ignored assists, blocks, and personal fouls. I also could not find any discussion of a position adjustment. That measure performs much worse than any other measure here.)
NBA Efficiency is is a lot like Wins Produced/Win Score - terrible without positon adjustments and good with them. In fact, if NBA efficiency is fixed so that similar efficiencies on two pointers and three pointers are counted the same, then NBA Efficiency with position adjustments is slightly better than Wins Produced/Win Score. This is contrary to what the authors argue in the book. Given how much of their argument in parts of their rests on their measure being better than NBA Efficiency (and not because of the position adjustments), these findings would significantly change their conclusions.
PER is much better than Wins Produced/Win Score without position adjustments, but once position adjustments are made, Wins Produced/Win Score does a little better than PER. But given that adjusting for position is much less important for PER, one might prefer PER on the grounds that positions are sometimes very difficult to determine for some players.
Dean Oliver's Offensive Rating minus Defensive Rating (at least I think this is Dean's) is worse than PER without position adjustments. But with position adjustments, it does a tiny bit better.
Win Shares comes out smelling like a rose. Without position adjustments it comes out better than any of the other measures so far. And unlike the other measure position adjustments actually make it worse, not better. Win Shares looks like it does not get as much credit as it should.
(Surprisingly, Win Shares per minute is correlated less with adjusted plus/minus ratings that regular Win Shares are.)
Finally, I included my statistical plus/minus metric, which does much better than any of the other measures. But that is not surprising, since it is designed to predict adjusted plus/minus ratings. Now I will not go into detail about how it is computed, but it does incorporate complicated adjustments for position and for other roles besides position. A really simple version of my statistical adjusted plus/minus measure without position adjustments has a correlation of 0.5380.
So we probably should not be so hard on Berri, Schmidt, and Brook as their measures do pretty well. There may be problems with the approach used to arrive at Wins Produced/Win Score, but once the position adjustments are included, they are pretty good metrics. These results are also a testament to Win Shares - doubly so since there is no need to position adjust with this measure.
Last edited by Dan Rosenbaum on Fri Jul 28, 2006 10:26 pm; edited 6 times in total |
|
Back to top |
|
 |
deepak
Joined: 26 Apr 2006 Posts: 665
|
Posted: Fri Jul 28, 2006 12:43 am Post subject: |
|
|
Thanks for this.
This is probably a silly question, but were you looking at Wins Produced, Win Scores, and Win Shares per possession? I know the rest are per-possession metrics, by definition.
Also, was the correlation to your adjusted +/- metric uniform across different types of players for each of the stats you looked at? For example, does PER do as good a job at estimating the effectiveness of guards as, say, big men? |
|
Back to top |
|
 |
Dan Rosenbaum
Joined: 03 Jan 2005 Posts: 541 Location: Greensboro, North Carolina
|
Posted: Fri Jul 28, 2006 1:01 am Post subject: |
|
|
deepak_e wrote: | Thanks for this.
This is probably a silly question, but were you looking at Wins Produced, Win Scores, and Win Shares per possession? I know the rest are per-possession metrics, by definition.
Also, was the correlation to your adjusted +/- metric uniform across different types of players for each of the stats you looked at? For example, does PER do as good a job at estimating the effectiveness of guards as, say, big men? |
I put Wins Produced and Win Score into per 40 pace-adjusted minutes units, so that in essence makes them per possession. I will add a Win Share measure that is per minute, which is actually worse than the regular Win Share.
The correlations do vary by position. PER struggles the most relative to the other metrics with 3s, but does well with 1s and 2s. Wins Produced struggles the most with 4s, but does well with 5s. At this point I am not sure why. |
|
Back to top |
|
 |
Mark
Joined: 20 Aug 2005 Posts: 807
|
Posted: Fri Jul 28, 2006 1:15 am Post subject: |
|
|
Very interesting discussion you are opening here. After I read your first post I composed a response but your second post addresses one of the main thrusts I was going to make so I will try to adjust to that.
Regarding the second post:
I wonder if you could try to include protrade in the method comparison.
Understanding the variances from actual wins by each method is worth doing and perhaps looking at multiple years would also be helpful.
The main task is understanding what goes into offensive and defensive efficiency. The different methods present a choice: should we just count the traditional stats, or try to count more things (spacing, double teams, picks, blockouts, early ball movement, tips, saves, etc.), or focus on team productivity and give equal shares to those on the court as a team, or split credit to one, several or all players by rule and judgment of actual involvement in the play or the success or failure of the play based on play by play or tape? Should it be done at team level or by player? I think there is merit for both. Perhaps it would be possible to also lightly or vigorously test to find the best score with a blend of methods.
Would the authors of each method agree to a true prediction before the coming season?
Regarding the first post:
“Then they adjust their Wins Produced measure so that, on average, it is equal for every position. There really is no basis for this adjustment”
This does seem like an important point of discussion. How should players and positions be weighted? On offense if not equal then by their roles and role fulfillment? (As you apparently did) Team specific or league average? On defense perhaps it could be done by the average quality of players at that position (for example to address SF weakness)?
“Lastly, they adjust using team defensive measures.”
Ultimately an ideal player assessment would capture individual, team and help defense accurately rather than rely total on team roll-up equally for all.
“One of the implications of this approach is that there is no room for credit to be given for shot creation…In my opinion, understanding the value of creating shots is perhaps the most important aspect of analyzing basketball statistics. “
I agree it is important but shot creation and quality of shot creation are different things and to be clear quality of shot creation is different than shot results. Quality of shot creation could perhaps be measured in this new era with 82games and synergy data by a player’s past FG% for a set of zones, and shot types, coverages, and timing and perhaps even various aspects of matchup that play.
Viewing some stats are indicators of superior basketball ability and inferring to current uncounted activities that affect results may have some promise and some peril. For a speicifc player some insights can be posited about uncounted activities that might be affected by similar skills and physical abilities and checked by tape study and extreme charting for amount of correlation. Going to inferring from one skill to another for a set of players would needed to carefully checked as well.
” And I have tended to find that the weights for these stats differ a lot from the logic-based approaches of Dean Oliver, John Hollinger, and Berri and his co-authors.”
The correlation of these stats with winning may vary from logic but are they really more important than logic suggests? Can we prove that? I am asking for degree of confidence on this, not rejecting. Is it those actions specifically that is more important or are they in turn correlated with hidden uncounted factors? Should we just weight the indicator stats more heavily or continue to uncover the hidden uncounted and score them separately?
From your adjusted +/- explanation: "points, rebounds, and assists, the marginal effect of an additional point, assist, offensive rebound, and defensive rebound is 1.08, 1.14, 0.78, and 0.11 points per 100 possessions, respectively. “
Compared to Berri it would appear that in your adjusted +/- work you found assists much more correlated with success than Berri weights and defensive rebounding less correlated than Berri weights. Can further discussion push any further on these longstanding topics of debate?
“Players who attempt lots of 3 points and free throws appear to be more valuable than players who specialize in two point field goal attempts. “
Makes sense but how much should TS% be adjusted from its straightforward logical weighting? Could teams high or low on these and their overal FG% somehow be used to guess at the extra weights for these type of shooting strength? Or is that stretching too far? Context matters and varies by team, details of the player mix and lineups used on the floor and opponent.
“…the results suggest that holding all of these other game statistics constant, players who play more minutes tend to help their team point differential. This result would be expected if coaches observe and reward contributions not picked up in game statistics (e.g. good defense) by playing those players more minutes. Note, however, that the coefficient is not huge. Holding the other game statistics constant, the difference between a 20 minutes per game player and a 40 minutes per game player is only 2.16 points per 100 possessions – about the same as an extra steal per 40 minutes.”
“In the future I hope to add height and age/experience to these regressions. It appears to me that looking at the results in many of the tables that young, inexperienced players tend to have lower pure adjusted plus/minus ratings than their game statistics would suggest. It seems that the young, inexperienced players may not contribute as much to their teams in ways not picked up by game statistics.”
Thanks for sharing this data and the impression and look forward to perhaps hearing more in the future. Validates on average the coaches eye. Indeed it would be interesting to see more splits: by position for a given coach, perhaps by different coaches of same team over several years, and perhaps also league average by position, role, age, body type, contract.
"So we probably should be spending more time talking about why Wins Produced does a better job than PER. "
It seems pretty straightforward that wins produced captures more of defense than PER and that may be a main reason it performs better.
Adjusted +/- appears to win because it seeks to capture the traditional stats and the hidden uncounted, a broader sweep than any of these others. And applies a lot of statistically sophisicated and basketball smart work in the analysis of the information.
I still wonder how protrade's variable to player credit system based on rule from play by play or an even further enhanced credit assignment system based on tape could do compared to adjusted +/-.
Last edited by Mark on Sat Jul 29, 2006 10:47 am; edited 10 times in total |
|
Back to top |
|
 |
Dan Rosenbaum
Joined: 03 Jan 2005 Posts: 541 Location: Greensboro, North Carolina
|
Posted: Fri Jul 28, 2006 1:32 am Post subject: |
|
|
Thanks for all of the great comments, Mark. I cannot promise that I will get to all of them, but over time (and keeping in mind that I can't reveal all of my tricks), I will see what I can get to.
Last edited by Dan Rosenbaum on Fri Jul 28, 2006 10:27 pm; edited 2 times in total |
|
Back to top |
|
 |
Mark
Joined: 20 Aug 2005 Posts: 807
|
Posted: Fri Jul 28, 2006 1:39 am Post subject: |
|
|
10-4. |
|
Back to top |
|
 |
deepak
Joined: 26 Apr 2006 Posts: 665
|
Posted: Fri Jul 28, 2006 2:36 am Post subject: |
|
|
I don't understand how per-minute Win Shares could do worse than raw Win Shares. Seems to go against the fundamental APBRmetrics principle that per-minute (or per-possession) stats are superior. Any thoughts on this? |
|
Back to top |
|
 |
Mark
Joined: 20 Aug 2005 Posts: 807
|
Posted: Fri Jul 28, 2006 2:43 am Post subject: |
|
|
I assume most or all of these methods can be made to fit tighter with the win-loss pattern if they included some summary measure of variabilty. It would be interesting to see how much better they get after this tweak and to compare the amount of variability in the results of the methods and the amount of impact of the variability factor in explaining results. GMs could by this method perhaps determine a little more exactly what type of adjustment to make?
(I assume in the method result comparison list all of the methods are fit to win-loss based on season averages.)
Last edited by Mark on Fri Jul 28, 2006 7:24 pm; edited 1 time in total |
|
Back to top |
|
 |
Dan Rosenbaum
Joined: 03 Jan 2005 Posts: 541 Location: Greensboro, North Carolina
|
Posted: Fri Jul 28, 2006 9:43 am Post subject: |
|
|
deepak_e wrote: | I don't understand how per-minute Win Shares could do worse than raw Win Shares. Seems to go against the fundamental APBRmetrics principle that per-minute (or per-possession) stats are superior. Any thoughts on this? |
Maybe Justin when he gets back from a three-day road trip will have some thoughts on this. I am hardly an expert on Win Shares, and my intution follows yours. |
|
Back to top |
|
 |
Dan Rosenbaum
Joined: 03 Jan 2005 Posts: 541 Location: Greensboro, North Carolina
|
Posted: Fri Jul 28, 2006 9:56 am Post subject: |
|
|
I took a look at how well the different measures correlate with offensive and defensive adjusted plus/minus ratings and those results were pretty interesting too.
The problem with PER is that it explains almost none of defense. It's correlation with offensive adjusted plus/minus is higher than my statistical plus/minus measure - although some of that is due to my measure being more than a measure of offense.
Wins Produced/Win Score - even without the team adjustments for defense - do a pretty good job on defense, but this is where my statistical plus/minus measure gains a lot of traction versus all of the measures. It is hard to assess defense with box score stats, but it is possible to do quite a bit better than the logic-based approaches do. I think what is going on is I am able to pick up a lot of the "uncounted" contributions on the defensive side that are correlated with box score stats. |
|
Back to top |
|
 |
Mark
Joined: 20 Aug 2005 Posts: 807
|
Posted: Fri Jul 28, 2006 11:52 am Post subject: |
|
|
The biggest uncounted in many methods is shot defense. Offensive rating - Defensive Rating and Win Shares also have it in their roll-up. Protrade's credit/blame system also covers it. (So does defensive tendex and cumulative tendex incorporating it. EWins doesnt but could still be added to the method comparison.)
Protrade could in my view be tweaked and improved- currently opponent made shot blame and missed shot credit surprisingly gets split equally. I missed this point before; this is similar to adjusted +/-'s equal handling of shot defense credit and blame. I can understand the philosophy that defense is a team endeavor that would be used to explain this equal scoring choice but I personally might go 2/3 credit or blame to direct defender and 1/3 to rest of team but maybe 50-50 would be ok too. I dont see why shotdefense shouldnt be fairly similar to scoring shooting- protrades only gives 70-75% of the credit or blame to the shooter not 100%. (Ideally if based on tape it would cover defensive switches accurately and to allow blaming the guy whose breakdown somewhere lead to the evental shot- some or a lot).
With regards to short creation, with game shot charts by player it would be possible if they were scored against 82games chart of player scoring by distance or exact zone or synergy's list by zone and type of shot to calculate the quality of shots taken based for each shot on the average historical FG% of that player from that spot with heavy contest, contest or no contest and then compiled the FG% and find the average expected FG%. (i.e. a corner 3pt shot has a player historical FG%, as does a wing 3pt shot-a different one- as does 17 footer, 10 footer, a layup, etc.)
You could then by comparing the shot creation average expected FG% to actual FG% add specificity to the old adage he or we "got good shots but didnt make them" or more fully appreciate good nights making a high rate of tough shots.
Adding fouls and free throws you could make the comparison shot creation expected TS% vs actual TS%. Or simply label shots taken good, fair and poor ones and be evaluated on that and the actual returns.
Dan, in general terms does the adjustment work you do on the +/- data by player give scores produced by this method awareness of game to game volatility and scoreboard/win impact and serve as an advantage in the comparison to the other methods which do not have that as scored? Or not? You have an edge over some by fully covering defense and arguably over all in capturing the impact of other uncounted activity associated with a player on/off by player but I wondered if this might be another source of your edge?
Last edited by Mark on Fri Jul 28, 2006 7:58 pm; edited 1 time in total |
|
Back to top |
|
 |
Dan Rosenbaum
Joined: 03 Jan 2005 Posts: 541 Location: Greensboro, North Carolina
|
Posted: Fri Jul 28, 2006 3:37 pm Post subject: |
|
|
Mark wrote: | Dan, in general terms does the adjustment work you do on the +/- data by player give scores produced by this method awareness of game to game volatility and scoreboard/win impact and serve as an advantage in the comparison to the other methods which do not have that as scored? Or not? You have an edge over some by fully covering defense and arguably over all in capturing the impact of other uncounted activity associated with a player on/off by player but I wondered if this might be another source of your edge? |
I am not sure what you mean by "game-to-game volatility." Of course, player productivity is volatile, but what our metrics capture is the average of that volatile productivity.
By using adjusted plus/minus ratings as a barometer, I am not saying that it necessarily is the best way to evaluate individual players. In lots of cases sample sizes are too small to get accurate adjusted plus/minus ratings. (Among players playing less than 500 minutes per season, the correlation between adjusted plus/minus ratings from year to year is practically zero.) But in theory the adjusted plus/minus rating should capture practically all of the contributions a players makes towards winning - not just those we happen to record in a box score.
So if we think of the adjusted plus/minus rating as being made up of the true impact of a player and a random component that isn't related to anything, then it should be a very good barometer of which of these box score stat based metrics does the best job measuring that impact. Because of the random component, the correlation will never be one, but we can still compare the relative correlations.
One last point. Adjusted plus/minus ratings are context specific. But so are box score statistics. Put Steve Kerr is a situation where he had to create most of his team's shots and his efficiency would likely fall like a rock - just like his adjusted plus/minus rating. |
|
Back to top |
|
 |
Mark
Joined: 20 Aug 2005 Posts: 807
|
Posted: Fri Jul 28, 2006 6:08 pm Post subject: |
|
|
I struggled with how to phrase the question a little but basically I was seeking reassurance that the method comparison was apples to apples, that is all averages and not adjusted for game to game volatility.
"Adjusted plus/minus ratings are context specific." I am trying to understand how much.
I wondered if the analytic process for adjusted +/- provided an opportunity to capture and adjust the player's result +/- (different from the raw input +/-) for game to game volatility in a way that the other methods hadnt gone thru but could with some form of tacked on volatility measure. I wondered if it is was fair or not to say that the adjustment process was capturing not just the average +/- but more about the impact of the "when" of the data: the strong and weak stretches of play, and how frequent they were, and how they were distributed over games and perhaps how they impacted win-loss.
I'll make one more try at explaining what I meant with an example:
Would three players otherwise exactly identical on team +/- season data score the same under adjusted +/- if one had a really outstanding game every 5 nights and 4 games modestly below the resulting average, one played exactly the same every time right to that same average, and the last one played 3 games modestly above the resulting average but 2 games further below the average?
Somewhat separate from the above talk, your adj. +/- method I believe gives extra weight to clutch time actions, which would be a perfectly legitimate, smart competitive advantage over almost all the other methods. It is a small numbers of plays but could have big impact in matching to win-loss depending how it is done. If another comparison was made for adjusted +/- with/without clutch weighting how much of the competitive advantage comes from that feature vs. capturing the hidden uncounted? If the other methods built in clutch weighting (as protrade also does) how much ground could they make up?
I offer these comments in the spirit of understanding more about the results of the method comparison and looking for best practices and urging wider adoption of them.
Last edited by Mark on Sat Jul 29, 2006 10:50 am; edited 1 time in total |
|
Back to top |
|
 |
Dan Rosenbaum
Joined: 03 Jan 2005 Posts: 541 Location: Greensboro, North Carolina
|
Posted: Fri Jul 28, 2006 9:02 pm Post subject: |
|
|
Mark wrote: | "Adjusted plus/minus ratings are context specific." I am trying to understand how much.
I wondered if it is was fair or not to say that the adjustment process was capturing not just the average +/- but more about the impact of the "when" of the data: the strong and weak stretches of play, and how frequent they were, and how they were distributed over games and perhaps how they impacted win-loss.
I'll make one more try at explaining what I meant with an example:
Would three players otherwise exactly identical on team +/- season data score the same under adjusted +/- if one had a really outstanding game every 5 nights and 4 games modestly below the resulting average, one played exactly the same every time right to that same average, and the last one played 3 games modestly above the resulting average but 2 games further below the average? |
These three players would get the same adjusted plus/minus rating. The only "when" I account for is that I weight each possession by the probability that an extra point would change the outcome of the game with playoffs counting more than the regular season.
Quote: | Somewhat separate from the above talk, your adj. +/- method I believe gives extra weight to clutch time actions, a perfectly legitimate, smart competitive advantage over almost all the other methods. It is a small numbers of plays but could have big impact in matching to win-loss. If another comparison was made for adjusted +/- with/without clutch weighting how much of the competitive advantage comes from that feature vs. capturing the hidden uncounted? If the other methods built in clutch weighting (as protrade also does) how much ground could they make up? |
I am not sure how to answer the question, but the weighting does matter. |
|
Back to top |
|
 |
|
|
You cannot post new topics in this forum You cannot reply to topics in this forum You cannot edit your posts in this forum You cannot delete your posts in this forum You cannot vote in polls in this forum
|
Powered by phpBB © 2001, 2005 phpBB Group
|