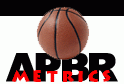 |
APBRmetrics The statistical revolution will not be televised.
|
View previous topic :: View next topic |
Author |
Message |
Dan Rosenbaum
Joined: 03 Jan 2005 Posts: 541 Location: Greensboro, North Carolina
|
Posted: Tue Feb 13, 2007 11:16 am Post subject: A Starting Point for Analyzing Basketball Statistics |
|
|
I moved this from the conference aftermath thread. We would love to hear any comments that folks have on this paper. We have tried to take many of the ideas developed in our community and bring them to the wider research community in this paper.
A Starting Point for Analyzing Basketball Statistics
(under review at the Journal of Quantitative Analysis in Sports)
http://www.uncg.edu/eco/rosenbaum/jqas1.doc
Abstract
The quantitative analysis of sports is a new branch of science and, in many ways one that has grown through non-academic and non-traditionally peer-reviewed work. The aim of this paper is to bring to a peer-reviewed journal the generally accepted basics of the analysis of basketball, thereby providing a common starting point for future research in basketball. The possession concept, in particular the concept of equal possessions for opponents in a game, is central to basketball analysis. Estimates of possessions have existed for approximately two decades, but the various formulas have sometimes created confusion. We hope that by showing how most previous formulas are special cases of our more general formulation, we shed light on the relationship between possessions and various statistics. Also, we hope that our new estimates can provide a common basis for future possession estimation. In addition to listing data sources for statistical research on basketball, we also discuss other concepts and methods, including offensive and defensive ratings, plays, per-minutes statistics, pace adjustments, true shooting percentage, effective field goal percentage, rebound rates, Four Factors, plus/minus statistics, counterpart statistics, linear weights metrics, individual possession usage, individual efficiency, Pythagorean method, and Bell Curve method. This list is not an exhaustive list of methodologies used in the field, but we believe that they provide a set of tools that fit within the possession framework and form the basis of common conversations on statistical research in basketball. |
|
Back to top |
|
 |
Ed Küpfer
Joined: 30 Dec 2004 Posts: 787 Location: Toronto
|
Posted: Tue Feb 13, 2007 11:19 am Post subject: |
|
|
Man, I've always wanted to do one of these. I'm glad not everyone is as lazy as me.
I just started reading. Is this in press already, or should I be watching for typos? _________________ ed |
|
Back to top |
|
 |
Dan Rosenbaum
Joined: 03 Jan 2005 Posts: 541 Location: Greensboro, North Carolina
|
Posted: Tue Feb 13, 2007 11:40 am Post subject: |
|
|
Ed Küpfer wrote: | Man, I've always wanted to do one of these. I'm glad not everyone is as lazy as me.
I just started reading. Is this in press already, or should I be watching for typos? |
It is only under review, so any comments - even on typos - are welcome. |
|
Back to top |
|
 |
Dan Rosenbaum
Joined: 03 Jan 2005 Posts: 541 Location: Greensboro, North Carolina
|
Posted: Tue Feb 13, 2007 11:44 am Post subject: |
|
|
HeatherA wrote: | Dan,
This is a great article. Thanks so much for sharing it. The extended work behind possession estimation alone makes it important reading.
Here's my question: you talk a lot in the paper about the limitations of various methods of individual player valuation (linear weights, plus/minus, counterpart statistics, etc.). I would be interested to know your thinking on which are the best tools currently out there for comparing players. Knowing that there is no perfect method of comparison (and that, perhaps we aren't even close yet), which of the measures that we do have available do you find more useful when attempting this type of work? |
I am not sure it is worth worrying about which method is "best." All of the methods provide a glimpse at a player and all of them are useful taken in context. Players don't produce "wins" directly. Players produce field goal attempts, picks, assists, spacing, one-on-one defense, post defense, help defense, etc. and every team has different needs for all of these qualities. Those differences arise from the other players on that team and on the system put in place by the coaching staff/front office. Players differ in the attributes they bring to a team, but good front office work/coaching is about finding players whose attributes will fetch the highest return for a given team.
In rare cases that might mean looking for the "best" player in some general sense, but more often such an approach is naive and misguided. It is an approach and mindset that is better suited for baseball than basketball. The market prices for different attributes must be balanced against the likely returns for those attributes on a given team. A scorer like Allen Iverson may not add a lot to an efficient offensive team like the current Phoenix Suns or Washington Wizards, but for a scoring-challenged team like the Sixers who went to the Finals, he likely was quite valuable.
Now if you were thinking about this for something general, like evaluations of college draft picks, then I don't think it really matters that much what you use. You could probably use minutes played and that would probably be a pretty good metric for that purpose. |
|
Back to top |
|
 |
HeatherA
Joined: 03 Aug 2006 Posts: 55
|
Posted: Tue Feb 13, 2007 12:46 pm Post subject: |
|
|
Dan Rosenbaum wrote: | HeatherA wrote: | Dan,
This is a great article. Thanks so much for sharing it. The extended work behind possession estimation alone makes it important reading.
Here's my question: you talk a lot in the paper about the limitations of various methods of individual player valuation (linear weights, plus/minus, counterpart statistics, etc.). I would be interested to know your thinking on which are the best tools currently out there for comparing players. Knowing that there is no perfect method of comparison (and that, perhaps we aren't even close yet), which of the measures that we do have available do you find more useful when attempting this type of work? |
I am not sure it is worth worrying about which method is "best." All of the methods provide a glimpse at a player and all of them are useful taken in context. Players don't produce "wins" directly. Players produce field goal attempts, picks, assists, spacing, one-on-one defense, post defense, help defense, etc. and every team has different needs for all of these qualities. Those differences arise from the other players on that team and on the system put in place by the coaching staff/front office. Players differ in the attributes they bring to a team, but good front office work/coaching is about finding players whose attributes will fetch the highest return for a given team.
In rare cases that might mean looking for the "best" player in some general sense, but more often such an approach is naive and misguided. It is an approach and mindset that is better suited for baseball than basketball. The market prices for different attributes must be balanced against the likely returns for those attributes on a given team. A scorer like Allen Iverson may not add a lot to an efficient offensive team like the current Phoenix Suns or Washington Wizards, but for a scoring-challenged team like the Sixers who went to the Finals, he likely was quite valuable.
Now if you were thinking about this for something general, like evaluations of college draft picks, then I don't think it really matters that much what you use. You could probably use minutes played and that would probably be a pretty good metric for that purpose. |
Of course. The system is complex, as are all systems. I totally agree.
I was, in fact, thinking more in generalities. Paul and I are doing some more historical player outcomes work and had started down the path of using NBA Efficiency as a starting place to get a handle on short-term "outcomes" (we realize that there are a host of things we need to control for here). The measure passed the initial giggle test when applied to players from 1980 on (sorted high to low, a rough eyeballing of the list didn't make us run in the other direction). After reading your paper, I started wondering whether we should be using a different metric.
If I read you correctly, it sounds like your general reaction is that any one of these metrics taken in isolation is flawed, but the linear metrics aren't particularly MORE flawed than the others.
Thanks for your thoughts. |
|
Back to top |
|
 |
Ed Küpfer
Joined: 30 Dec 2004 Posts: 787 Location: Toronto
|
Posted: Tue Feb 13, 2007 1:09 pm Post subject: |
|
|
HeatherA: Keep in mind that there's two types of player metric, production (eg Pts/G) and efficiency (eg FG%). Attempts to combine these are problematic. Because (as noted in the paper under discussion) the precise relationship between production and efficiency is unknown -- and largely unexplored -- I always look at both. Always.
NBA EFF, from what I recall, combines both. Yuck. For production, you'd do better, as a start, to regress team points on the various component stats (on a season-by-season basis) and apply that to the players. These so-called linear weights have a bad reputation among us statheads, but they should be adequate for your needs. Better still is to go with Dean Oliver's individual points per possessions, as outlined in his book. He writes about a Points Produces concept that would be useful.
As far as efficiency, you can also that individual points per possessions. For most players, EFG% and TS% pretty much captures most of the variance in ORTG, so if you want to go simple, you can use that. Add turnovers to the denominator if you want.
Another approach is to ignore composite metrics altogether and look solely at the component stats: REB%, EFG%, etc. The advantage of this is that we are much more confident that these metrics actually measure what we think they do. Also, these measure things that map closely to player skills we find interesting. The down side is that analysis is more complicated since you have increased the number of response variables.
I prefer the last approach. I'd rather know that a player is a good rebounder, poor shooter, great foul-drawer, etc, than know that he contributes an above average number of points per possession. That's just me, though. _________________ ed |
|
Back to top |
|
 |
HeatherA
Joined: 03 Aug 2006 Posts: 55
|
Posted: Tue Feb 13, 2007 1:31 pm Post subject: |
|
|
The NBA Efficiency stat is definitely a kitchen-sink kind of stat:
compute efficiency = ((Pts + Reb + Asts + Stl + Blk) - ((fga - fgm) + (fta - ftm) + turnover))
And I would never consider it if I were trying to do any serious analysis of individual players. However, my purpose is to get a more general sense of "player performance" for a really stat-lite general article.
When I calculate NBA Efficiency for players' rookie years 1980-2005, the top 20 players come out to be:
David Robinson
Kevin Garnett
LeBron James
Michael Jordan
Shaquill O'neal
Shawn Marion
Elton Brand
Kobe Bryant
Dirk Nowitzki
Dwyane Wade
Tim Duncan
Allen Iverson
Terry Cummings
Hakeem Olajuwon
Yao Ming
Larry Johnson
Chris Bosh
Paul Pierce
Alonzo Mourning
Gilbert Arenas
Eyeballing the list as a whole seems to make some sense as well. So I have some comfort that, while not by any means perfect, this stat is telling me something about players' relative performance in the NBA.
I like your thought about breaking down the individual pieces and looking at them separately, as well. I'll have to think about whether there is a way to include that without making the article completely unwieldy.
Thanks,
Heather |
|
Back to top |
|
 |
tpryan
Joined: 11 Feb 2005 Posts: 100
|
Posted: Tue Feb 13, 2007 6:33 pm Post subject: |
|
|
Dan,
First of all, congratulations on putting the paper together.
One comment I have is that the first sentence of your abstract creates the impression that quantitative analysis of sports is new, or at least a new branch of science. Certainly it is now being taken to a new level in professional basketball, but Bud Goode was modeling NFL games almost 50 years ago. In the peer-reviewed literature, David Harville had a paper on using linear model methodology for ranking college football teams in the Journal of the American Statistical Association in 1977 and many other papers have appeared in leading statistics journals.
And sophisticated statistical analyses have been applied to various other sports for many years.
I assume there are some moderately high correlations among the 7 variables in Table 1, so why not do the following.
There is now a routine in R (the freeware version of S-Plus for anyone not familar with it), that uses 6 different methods for assessing the relative importance of each variable in a regression model when the variables are correlated (see http://www.jstatsoft.org/v17/i01/v17i01.pdf ). Why not use this and compare the results with your Table 1? |
|
Back to top |
|
 |
Chronz1
Joined: 22 May 2006 Posts: 201
|
Posted: Tue Feb 13, 2007 6:45 pm Post subject: |
|
|
Just a question, why would anyone use NBA EFF when PER is like the upgraded form that adjusts for pace and minutes played. |
|
Back to top |
|
 |
HoopStudies
Joined: 30 Dec 2004 Posts: 705 Location: Near Philadelphia, PA
|
Posted: Tue Feb 13, 2007 7:13 pm Post subject: |
|
|
tpryan wrote: |
And sophisticated statistical analyses have been applied to various other sports for many years.
|
Science has, of course, been applied to sports before, but sports have not really been considered a science on its own until very recently. Applications of stats to sports has been around, but I'd say is distinct from Quantitative Analysis of Sports. Baseball and basketball and football have developed significant groups of people (like us) interested in analyzing the sports with whatever quantitative tools exist, integrating traditional knowledge. It is that group of work that has built the foundations of the science, not the isolated papers on sports that exist in psych, econ, management, etc. journals. Dave Berri has acknowledged that he built his methods to help them do economics, not to build a science of sports. What we outline in the paper is to illustrate that the science of basketball has been built, is pretty solid, and can (thanks to JQAS) exist in a peer-reviewed pub.
tpryan wrote: |
I assume there are some moderately high correlations among the 7 variables in Table 1, so why not do the following.
There is now a routine in R (the freeware version of S-Plus for anyone not familar with it), that uses 6 different methods for assessing the relative importance of each variable in a regression model when the variables are correlated (see http://www.jstatsoft.org/v17/i01/v17i01.pdf ). Why not use this and compare the results with your Table 1? |
Actually, that package is not available to US users so we can't use it. It does sound quite interesting. _________________ Dean Oliver
Author, Basketball on Paper
The postings are my own & don't necess represent positions, strategies or opinions of employers. |
|
Back to top |
|
 |
Dan Rosenbaum
Joined: 03 Jan 2005 Posts: 541 Location: Greensboro, North Carolina
|
Posted: Tue Feb 13, 2007 8:26 pm Post subject: |
|
|
tpryan wrote: | I assume there are some moderately high correlations among the 7 variables in Table 1, so why not do the following.
There is now a routine in R (the freeware version of S-Plus for anyone not familar with it), that uses 6 different methods for assessing the relative importance of each variable in a regression model when the variables are correlated (see http://www.jstatsoft.org/v17/i01/v17i01.pdf ). Why not use this and compare the results with your Table 1? |
I think Dean makes an important clarification of what we were trying to say in the abstract, but if you think the word "new" is likely to needlessly offend folks, I don't think we are married to that word.
Correlation is not a problem in our regression model. The standard errors are microscopically tiny, so we have plenty of variation to identify the effects of each of the variables. More importantly, we have solid theoretical reasons for believing that each of these variables in (1) belong in the model, so we don't have any need to model search. And the empirical results strongly support our theory. We estimate (2) just to show what happens when we limit the variables to those from the offensive team as a matter of convenience. |
|
Back to top |
|
 |
HeatherA
Joined: 03 Aug 2006 Posts: 55
|
Posted: Tue Feb 13, 2007 9:50 pm Post subject: |
|
|
Chronz1 wrote: | Just a question, why would anyone use NBA EFF when PER is like the upgraded form that adjusts for pace and minutes played. |
We're looking at over two decades of historical data. Pace would not be easy to ascertain. |
|
Back to top |
|
 |
Neil Paine
Joined: 13 Oct 2005 Posts: 774 Location: Atlanta, GA
|
Posted: Tue Feb 13, 2007 10:17 pm Post subject: |
|
|
HeatherA wrote: | Chronz1 wrote: | Just a question, why would anyone use NBA EFF when PER is like the upgraded form that adjusts for pace and minutes played. |
We're looking at over two decades of historical data. Pace would not be easy to ascertain. |
Pace Factor can be calculated for every season since 1974, and Justin even has a semi-reliable way of making pace adjustments for every season since 1952. |
|
Back to top |
|
 |
mtamada
Joined: 28 Jan 2005 Posts: 377
|
Posted: Tue Feb 13, 2007 10:48 pm Post subject: |
|
|
HoopStudies wrote: | tpryan wrote: |
There is now a routine in R (the freeware version of S-Plus for anyone not familar with it), that uses 6 different methods for assessing the relative importance of each variable in a regression model when the variables are correlated (see http://www.jstatsoft.org/v17/i01/v17i01.pdf ). Why not use this and compare the results with your Table 1? |
Actually, that package is not available to US users so we can't use it. It does sound quite interesting. |
Well the article says that one of their recommended metrics, "pmvd", is not available in the US (due to potential patent problems), but the other one, "lmg", is available.
DanR is correct however, with a model based on theory, one builds the variables directly into the model rather than waiting for the regressions to say what variables appear to be important. |
|
Back to top |
|
 |
tpryan
Joined: 11 Feb 2005 Posts: 100
|
Posted: Tue Feb 13, 2007 11:35 pm Post subject: |
|
|
Quote: | Well the article says that one of their recommended metrics, "pmvd", is not available in the US (due to potential patent problems), but the other one, "lmg", is available. |
Yes, there are two versions of the program and one is available in this country and can be downloaded at http://CRAN.R-project.org.
Quote: | DanR is correct however, with a model based on theory, one builds the variables directly into the model rather than waiting for the regressions to say what variables appear to be important. |
I wasn't thinking about variable selection. Rather, I was thinking about the paragraph above Table 1 in which the coefficients are interpreted. The standard errors are probably small because there is a very large amount of data, but that doesn't mean that the coefficients can be interpreted as giving the relative possession values of the variables.
I would use appropriate software to try to get a handle on the latter.
In addition to the program we are discussing that can be downloaded at the R project website, there is also a program (hierpart) in R that implements the hierarchical partitioning algorithm of Chevan and Sutherland. If you google "Chevan Sutherland partitioning software", you will see a number of papers in which the method and software have been used. |
|
Back to top |
|
 |
|
|
You cannot post new topics in this forum You cannot reply to topics in this forum You cannot edit your posts in this forum You cannot delete your posts in this forum You cannot vote in polls in this forum
|
Powered by phpBB © 2001, 2005 phpBB Group
|